One goal of GEOD@C is to improve data literacy among participants. We seek to improve how well participants are able to obtain, transform, analyze, and produce data sets. This skillset is highly desired in technology, engineering, and research firms.
Why do we approach learning data skills the way we do? GEOD@C aims to foster a “convergent” and “engaging” learning environment by being active, inclusive, and collaborative. Imagine you measure the skill level of everyone participating in a learning activity at the start and again at the end. The activity reshapes the distribution of skill levels among participants – some subset of participants is going to improve! But the learning environment dictates how the shape changes. We will explore three possible changes.
Lowest Common Denominator: Convergent but not Engaging
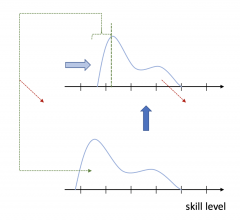
Change in skill level in lowest common denominator activity
The lowest common denominator approach to learning is a good example. Here, we define a standard level, sometimes using a standardized test, and the learning activity is designed to get everyone above that standard. The standard itself serves as the motivation, because it is a barrier to further progress. Participants who fail the test might need to try the activity again. Lowest common denominator learning shifts the lower skill levels in the distribution. Participants who enter at a higher skill level might find themselves left to their own devices. Thus, progress at the higher end of the skill pool is small. Worse still, participants at both ends can lose interest and cease the activity. If the goal of the activity is to increase the overall skill level, then people leaving the activity due to boredom (high initial skill) or discouragement (low initial skill) is a failure of the activity. This learning environment might be “convergent” (the distribution of skill levels after the activity gets narrower), but it is not “engaging”.
Performance-based: Divergent
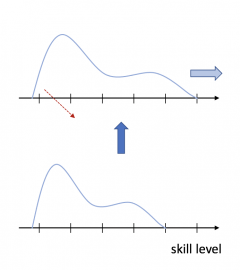
Change in skill level in divergent learning mode activity
A typical performance-based hackathon provides a different example. In this case, the best performers receive awards. Participants who enter at a high skill level are already likely to earn these awards, and so will strive to deliver their best work. Those with lower initial skill levels may find themselves unmotivated by awards that are beyond their reach. This creates divergence in the skill distribution: those at the high end might improve while those at the low end remain the same. Again, there is a risk of people leaving the activity due to a lack of motivation. The learning environment is “divergent” and still not “engaging”.
Our Ambition: Convergent and Engaging
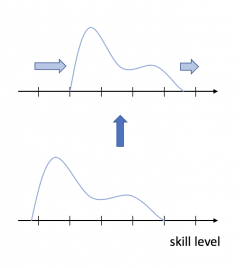
Change in skill level in a convergent, engaging learning activity
The goal with GEOD@C’s code nights is to create a convergent shift in the skill level of participants. We also want to create progress at both the high and low initial skill levels. By using a motivation that is inclusive and independent of initial skill level (for example, all participants in the activities are included in the challenge dataset’s authorship), we hope to maintain interest within all levels of participants. Further, we structure the code night activities so that they appeal to many skill levels, with tiered achievements. Finally, the learning activity is active: we engage participants in building a dataset with a practical use and a life beyond the activity.