Katherine Davidson is a PhD candidate in the Department of Sociology and Anthropology
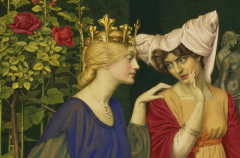
Detail of ‘Changing the Letter’, 1908, by Joseph Edward Southall; shared on Unsplash.com by The Birmingham Museums Trust
For those of us using audio recording for data collection – one-on-one or group interviews, archival audio, collaborative audio-visual methods – having a good resource for accurate and fast transcription is a game-changer.
Transcription services quickly turn your audio or audio-visual data into text files to be used in your research, like a transcript of The Payback Museum from Last Week Tonight which relates to our work studying illicit trade networks, or a number of revolutionary speeches as translated by Dr. Graham here (https://electricarchaeology.ca/2022/09/22/whisper-from-openai-for-transcribing-audio/). Having this data in a text format is handy for reading and editing data collected from conversations, and written text can also be fed into a number of models and graphs to help analyze discussions. Transcription services will also often output into formats that you can use for captioning, which makes your data more accessible to the public, in particular to folks with hearing impairment or auditory processing disorders.
My doctoral research involves conducting workshops around object elicitation (using artifacts as a memory trigger for discussions about the past), and the way I am capturing the discussions is through audio recordings during the workshops. With ~9 hours of audio recordings from my first workshop, AI transcription gives me a great place to start going through these conversations to pick out themes, main points of interest and questions from participants.
AI transcription makes the heavy lifting of writing out hours and hours of audio a breeze, though you should still go through the transcription alongside the audio and verify it. This is the bit that takes me a long time – pausing and annotating, or re-listening to a quip that I didn’t quite hear correctly. Having a clear audio recording makes transcription a lot easier to discern, both for the researcher and AI – I wish I had known that sooner!
Now, having tried a few different transcription resources to transcribe hours of audio from fieldwork for my doctoral thesis, here are a few recommendations based on what I’m liking now. I am very pleased with the accuracy of Whisper AI (not to mention, it’s free!). I also really like Trint’s (https://trint.com/) infrastructure for listening to audio and highlighting the current place in the text, but it’s not as accurate as Whisper AI, and it’s fairly expensive for a student budget.
For those of you looking to use Whisper AI, there is a user-friendly Google Colab notebook available at our Xlab repo here: https://github.com/XLabCU/useful_notebooks. This will walk you through linking your Google Drive to input/output audio files, as well as translate between different languages. For the output model size, we have found the “medium” size to be a good combination of accuracy and speed.
Pro tip: if you find a python notebook file, .ipynb, on Github that you want to run in Google Colab, in the address bar of your browser remove the ‘https://github.com/’ part of the address and replace with ‘https://colab.research.google.com/github/’; thus with our Whisper notebook, you can run it directly at this address: https://colab.research.google.com/github/XLabCU/useful_notebooks/blob/main/Google_Colab_Notebook_for_Transcription_using_Whisper_AI.ipynb . Cool, eh?