Jonah Ellens is a second year student in the Department of History
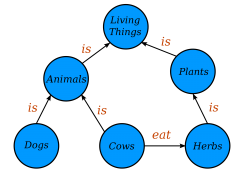
Conceptual Diagaram, by Jayarathina on Wikimedia Commons, CC BY-SA 4.0
This year I’m continuing to assist Xlab’s ‘The New Organigram Project‘ as a researcher. For the Organigram Project uses machine learning to help build a knowledge graph modelling the trafficking of stolen antiquities. To support the work, I get to delve into the exciting world of machine learning and knowledge graphs to see what creative ideas people are coming up with to solve problems.
Machine Learning
There are a lot of exciting articles describing the recent work using machine learning to build knowledge graphs. Machine learning technologies are easily available today and are constantly being developed into more powerful tools. One of the tasks we are interested in teaching computers to perform is Named Entity Recognition (NER). More simply, it’s a operation that allows a machine to process the language we use in everyday communication so that it can do something useful with the information. Computers are already effective at finding specific words a mass of text but it is more useful if they can understand those words within the context of a sentence to refine the search. A common application for NER is to use programs to search for relevant information in large databases of text.
Knowledge Graphs
Knowledge graphs are bodies of interconnected ideas. They attach information together but the relations are just as important as the nodes being connected. It’s like a web but each of the threads carry information as well. For example, person A and person B are separate nodes but they can be connected by relations of teacher and student. This allows us to focus on how and why entities are connected together to create more informed networks out of data.
My Work
In the course of research for the Organigram project, I look at similar work to see what problems people are using machine learning and knowledge graphs to solve and how they combine and train software to accomplish their goal.
Some exciting examples of what I’ve found show that these technologies can be applied to very specific tasks. Heritage Connector is a knowledge graph of science museum collection records created using machine learning. This allows researchers to search through millions of items belonging to several museums. The advantage is that it is relatively easy to run and requires little preparation for museums to contribute their own files. This makes it easier for small museums, known for possessing small budgets and little spare time, to join the program a make their resources more accessible.
Another example of machine learning is ArcheoBERTje, a NER search engine trained to recognize archaeology terms in Dutch records. Instead of running a keyword search of metadata, the program can find words in the text consisting of decades of reports. This allows researchers to make searches for specific artifacts from sites excavating different eras with less greater precision.
Conclusion
I find this work exciting because it explores projects were people and technology work well together. The computers can process basic data quickly while us humans lend our understanding of complicated and nuanced communication. I’m looking forward to see how these technologies change the way we do our research in the future.