Samir Khan is the Director of Research with the US-based organization GivingTuesday, which inspires hundreds of millions of people around the world to give, collaborate and celebrate generosity. In Canada, $16 million was raised on GivingTuesday (Dec. 3, 2024), and in the US, $3.6 billion was donated on the same day. He spoke to PANL Perspectives about what his organization is doing in terms of data collection and tools – and its research and use of generative AI. He also shared an overview and a walkthrough of Data Commons projects: GivingTuesday Data Commons – Overview for Academic Researchers (Oct 2024) and GivingTuesday Data Commons – Asset Walkthrough, which reviews about a dozen Core Date Projects and Special Projects
Question: What is GivingTuesday doing in terms of data related to the nonprofit sector?
Samir Khan: GivingTuesday is creating better data, better tools and better insights about what’s going on in our sector — from individuals’ and institutional behaviours to trends in the nonprofit sector to how the social sector, writ large, is being resourced. This includes working with networks that aren’t government and aren’t nonprofits, such as Kickstarter and social enterprises.
Our research initiatives are held together by a research agenda that covers three topics:
- understanding how resources are mobilized in the social sector;
- understanding how people feel and what they do in terms of support work in their communities; and
- understanding GivingTuesday as a lynchpin for diverse actors who work together on community participation and resource mobilization.
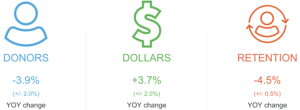
GivingTuesday’s Data Commons contains the Fundraising Effectiveness Project (FEP), which structures private data for a sectoral-eye view and is available for free to the public. For example, in the second quarter of 2024, GivingTuesday tracked a 3.7% increase in dollars raised in the US, while the number of donors and donor retention fell by -3.9% and -4.5%, respectively.
For instance, Data Commons provides infrastructure and research technology that enables the collection, exploration and sharing of data wherever it might exist. So, we’re partly a think tank, partly a technology service, and partly a complex series of relationships with the public and private sectors. We’ve created platforms such as the “990 Data Infrastructure Project” and the Fundraising Effectiveness Project (FEP) – and when you go to Data Commons, you’ll see a bunch of other free survey data and insights that are readable and usable for research.
Q: Which data is Canadian and which, global?
Samir Khan: We have annual surveys about giving behaviour in Canada — something called the Global Omnibus Survey, which has about three years of one-year snapshots of data. These were highlighted most recently in “The Giving Bridge: A Lookback at 2023 Trends in Global Generosity,” which investigates the nature of generosity globally using datasets. In Canada, as in the US, we see the same trend: a large group of people give in multiple ways.
We also house and curate datasets about giving from around the world – through hubs. In addition to the United States and Canada Hub, the GivingTuesday India Hub supports research about the “everyday giving market,” which looks at what Indians do to support causes and issues they care about. Also, we’re forging partnerships in the Latin American and the Caribbean Hub, with research institutes there, to do similar types of projects.
Q: What work are you most proud of at GivingTuesday – or what stands out for you?
Samir Khan: In what feels like an increasingly fragmented and polarized time, what’s impressive to me is the data showing large numbers of people participating, donating and supporting charitable work in their communities – across many demographics. A lot of people worry about the slow decline in numbers of people participating and donating, compared to 20 years ago, but I still think there’s a lot more strength in community-purpose and social-purpose organizations than people think there is.
Q: Do you track or collect data related to Kickstarter, GoFundMe or other crowdfunding platforms?
Samir Khan: In datasets, we can see how many people are giving to individuals and can understand a bit about whether that giving is being mediated by one of those platforms. One thing that we’ve found in our datasets is that, in aggregate, people who give one way tend to give in a whole bunch of different ways to both individuals and formal nonprofits – and they tend to volunteer time as well.
Some people might say, “Supporting causes through crowdfunders is wrong, because of verification problems,” but it’s worth asking about those donors’ motivations and connections, especially because crowdfunders have evolved with new technologies. There’s probably a lot more to learn from that giving behaviour than to be fearful of.
Q: Is GivingTuesday using generative AI tools – or researching the topic of new AI tools?
Samir Khan: We published the global results of an AI readiness survey, “Where Are We Now? AI Readiness and Adoption in the Nonprofit Sector in 2024.” It wasn’t a representative sample, but we learned that people are using generative AI to be more productive, such as creating a good first draft of something that they can edit — and that saves them time. But I think, you know, in 10 years, we’ll look up and ask ourselves if all that investment in AI was needed, whether the benefits were worth it. It’s an exciting time, assuming people know the limitations of the technology, such as “hallucinations.”
Our data science team has embraced AI in creative ways, using large language models (LLMs) to help them parse certain types of datasets – like qualitative datasets or plain-text datasets. The goal is to get AI to do more grunt work more quickly. Many folks are also using it to help them to generate first ideas for certain types of code that they know that they have to write, code that’s relatively predictable.
In terms of statistical modeling, though, I haven’t seen examples where AI has been able to do rigorous regression analysis. Maybe some of your readers or viewers will correct me. This is in the realm of social science research. I won’t speak about its applications for medical research, which I know a lot of people are excited about. Essentially, AI hasn’t been able to show evidence of reasoning that a human researcher would apply in a statistical or in a regression-analysis context. That’s my understanding of it. I might be wrong by the time this goes up!
Monday, December 16, 2024 in General
Share: Twitter, Facebook